Businesses face decision-making challenges due to unpredictable market trends. Predictive analytics can assist, as relying on historical data risks mismanaging inventory, finances, and customer relations. This outdated reliance increases the likelihood of reduced productivity and financial losses.
Tiyak na hindi mo gustong mangyari iyon sa iyong negosyo.
When integrated into ERP software, predictive analytics transforms decision-making by analyzing data patterns to forecast outcomes. It helps businesses optimize inventory, improve financial planning, and enhance customer engagement while providing real-time insights for proactive risk management.
Curious about how predictive analytics can revolutionize business operations? Stay tuned to discover its impact on efficiency, growth, and long-term success.
Table of Content
Content Lists
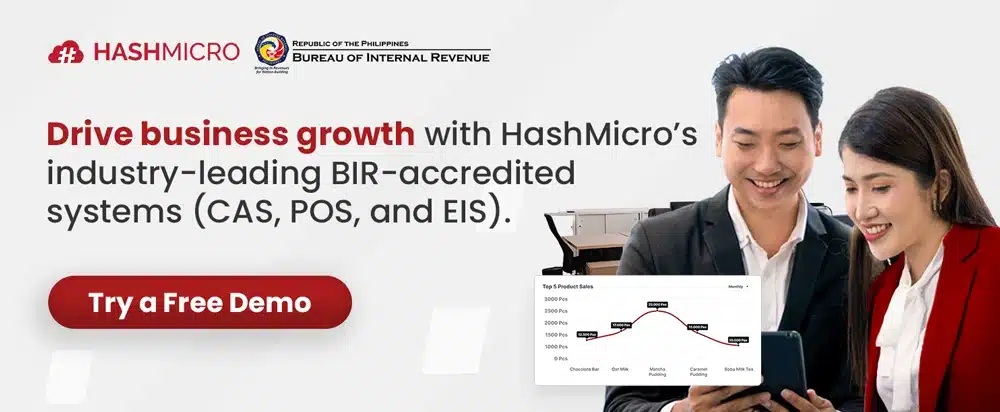
Key Takeaways
[wpcode id=”14816″ text=”Click Here to Get the Free Demo!”] |
What is Predictive Analytics?
Predictive analytics is a data-driven approach that uses statistical techniques, machine learning, and historical data to forecast future trends and outcomes. It helps businesses anticipate potential challenges, optimize decision-making, and improve operational efficiency.
Predictive analysis is widely adopted across various business scales to enhance decision-making and improve efficiency. Large enterprises use predictive analytics for supply chain optimization, financial forecasting, and resource planning.
Use of Predictive Analytics across Businesses
Predictive analytics is utilised across industries to improve decision-making and operational efficiency. Therefore, focusing on the key applications of predictive analysis is essential. What are they? Here are some significant applications of predictive analysis:
1. Demand forecasting
Businesses use predictive analytics to anticipate customer demand, helping optimize inventory levels and supply chain operations. It enhances demand forecasting by leveraging historical data, identifying patterns, and using machine learning to predict future demand.
2. Fraud detection and risk management
Financial institutions and insurance companies rely on predictive analytics to identify fraudulent transactions and assess credit risks. It helps detect anomalies in economic activities, reducing potential losses.
3. Customer insights and personalization
Companies analyze customer behavior to create personalized marketing campaigns and improve customer retention. Businesses can enhance engagement and boost sales by predicting preferences and purchase patterns.
4. Predictive maintenance
Manufacturing and logistics companies employ predictive analytics to monitor equipment performance and prevent failures. A smart warehouse analyzes historical data and real-time sensor inputs to detect potential issues before disruptions occur.
5. Workforce and HR planning
HR departments leverage predictive analytics to forecast employee turnover, optimize hiring strategies, and improve workforce management. It helps organizations ensure better talent retention and operational stability.
Types of Predictive Analytical Models
Predictive analytics relies on various models to analyze data, identify patterns, and forecast future outcomes. These models help businesses optimize decision-making, reduce risks, and improve operational efficiency. Below are the key types of predictive analytical models.
1. Regression models
Regression model identifies relationships between variables to predict values like sales forecasts and revenue trends. Businesses use regression models to estimate future outcomes from historical data, aiding financial planning and market analysis.
2. Classification models
These models categorize data into groups, aiding businesses in fraud detection, customer segmentation, and risk assessment. Financial institutions use them to assess loan eligibility, while healthcare providers use them for disease diagnosis.
3. Decision tree models
A decision tree model is a flowchart that predicts outcomes using decision rules. It simplifies decision-making by breaking choices into logical steps. Businesses use decision trees to assess customer churn, evaluate risks, and optimize strategies.
4. Clustering models
Clustering models aid customer profiling, market segmentation, and supply chain optimization by grouping similar data points. Retailers categorize customers by purchasing behaviors, while logistics companies optimize delivery routes.
5. Time series models
A time series model analyzes data over time. It is commonly used for demand forecasting, stock predictions, and sales trends. Businesses use it to anticipate demand fluctuations, adjusting production schedules and resource allocation accordingly.
6. Neural networks and machine learning models
These advanced models simulate human decision-making by analyzing large datasets and identifying complex patterns. They are used in AI analytics, allowing businesses to automate processes, detect anomalies, and enhance forecasting accuracy.
Benefits of Predictive Analytics
Predictive analytics empowers businesses with data-driven insights, allowing them to make informed decisions and improve overall efficiency. Below are the key benefits of implementing predictive analytics.
1. Enhanced decision-making
By analyzing historical and real-time data, businesses can make accurate, data-driven decisions. Predictive analytics reduces guesswork and ensures strategic planning based on reliable insights.
2. Improved operational efficiency
Predictive analytics helps identify inefficiencies and optimize workflows, reducing bottlenecks and enhancing productivity. Companies can streamline inventory management, production planning, and resource allocation.
3. Risk mitigation
Businesses can anticipate risks, such as financial losses, fraud, or supply chain disruptions, and take preventive measures. This proactive approach minimizes uncertainties and protects business stability.
4. Optimized supply chain management
Forecasting demand and tracking trends can help businesses improve supply chain management, reduce waste, and ensure timely goods delivery. It is particularly beneficial in industries with fluctuating market demands.
5. Increased customer satisfaction
Predictive analytics enables businesses to understand customer behavior, personalize marketing strategies, and improve customer experiences. By anticipating needs and preferences, companies can enhance engagement and loyalty.
6. Cost reduction
Through accurate forecasting, businesses can reduce unnecessary expenses, prevent overstocking or understocking, and optimize resource utilization. Predictive analytics leads to better budget management and increased profitability.
7. Competitive advantage
Companies that leverage predictive analytics can stay ahead of market trends, respond faster to changes, and make smarter strategic moves. It helps them maintain a strong position in an increasingly data-driven business environment.
Predictive Analytics Examples
Predictive analytics is widely applied across various industries to improve efficiency, mitigate risks, and enhance decision-making. Here are some real-world examples of how businesses use predictive analytics.
1. Retail: Personalized customer recommendations
E-commerce platforms and retail businesses use predictive analytics to analyze past purchase behavior and browsing history. By identifying customer preferences, companies can provide personalized product recommendations, increasing conversion rates and customer satisfaction.
2. Finance: Fraud detection and risk assessment
Banks and financial institutions utilize predictive analytics to detect fraudulent transactions by analyzing spending patterns and flagging anomalies. It also helps assess credit risk by evaluating a customer’s economic history and predicting the likelihood of loan defaults.
3. Manufacturing: Predictive maintenance
Manufacturers leverage predictive analytics to monitor equipment performance and predict potential failures before they occur. Predictive analysis helps reduce unplanned downtime, lowers maintenance costs, and improves operational efficiency.
4. Supply Chain: Demand forecasting and inventory optimization
Companies use predictive analytics to forecast future demand trends, ensuring optimal inventory levels. It prevents overstocking or shortages, leading to better supply chain management and cost savings.
5. Marketing: Customer churn prediction
Businesses analyze customer interactions and engagement levels to predict which customers are likely to stop using their services. Companies can implement targeted retention strategies to improve loyalty by identifying at-risk customers.
Features to Look for in Predictive Analytics Tools
Selecting the right predictive analytics tool is essential for businesses to maximize efficiency and make accurate, data-driven decisions. With various options available, companies should consider the following key features to ensure the tool aligns with their needs.
1. Scalability and performance
A reliable predictive analytics tool should be able to handle large datasets and grow with the business. Scalability ensures the system maintains high performance without lagging as data volume increases.
2. Integration capabilities
The tool should seamlessly integrate with existing business software, such as ERP systems, CRM platforms, and BI tools. Strong integration capabilities allow for smooth data flow across departments, enhancing decision-making.
3. User-friendliness and accessibility
A well-designed interface with low-code or no-code capabilities ensures that technical and non-technical users can effectively leverage predictive analytics. Intuitive dashboards and automated workflows improve usability across teams.
4. Real-time data processing
Businesses need predictive analytics tools that provide real-time insights, enabling them to react quickly to market trends, supply chain disruptions, or customer behavior shifts. Real-time data enhances agility in decision-making.
5. AI and machine learning support
Advanced AI-driven analytics improve prediction accuracy and automate complex data modeling. Machine learning capabilities enable continuous learning, ensuring that forecasts become more precise over time.
6. Data security and compliance
Since predictive analytics tools process sensitive business data, security measures such as data encryption, access controls, and compliance with industry regulations (e.g., Data Privacy Act of 2012 (Republic Act No. 10173)) are crucial to protect information integrity.
7. Customizability and flexibility
Every business has unique needs, so predictive analytics tools should allow customized reports, adjustable forecasting models, and adaptable data visualization options to meet specific operational goals.
Choosing the right predictive analytics tool is vital for businesses aiming to improve decision-making and efficiency. We provide a pricing scheme detailing our solutions’ costs and features. Download it now to explore the best options for your business.
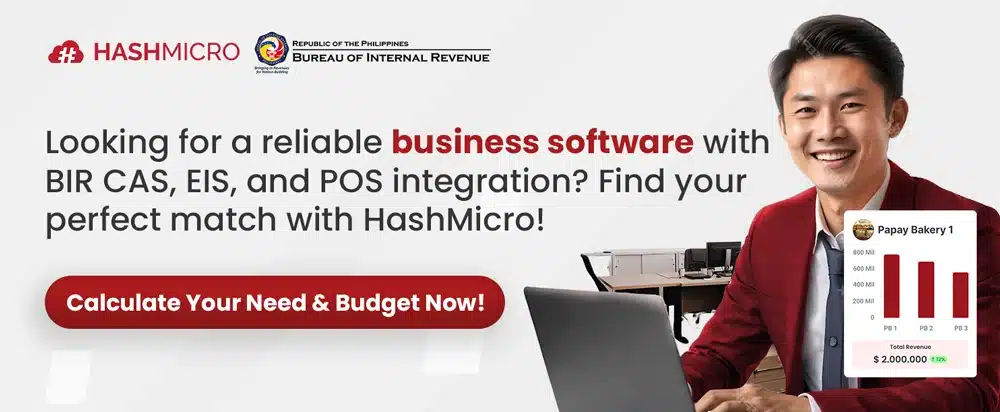
How Businesses Can Use Predictive Analytics
Implementing predictive analytics requires a strategic approach to ensure businesses maximize the benefits. Below are key steps to successfully integrate predictive analytics into business operations.
1. Define business objectives
Identify specific goals, such as improving sales forecasts, reducing operational risks, or enhancing customer retention, to align predictive analytics with business needs.
2. Collect and organize data
Gather high-quality, relevant data from multiple sources, including sales records, customer interactions, and market trends. Ensuring data accuracy is crucial for reliable predictions.
3. Choose the right predictive model
Based on business requirements, select appropriate models, such as regression for sales forecasting, classification for fraud detection, or decision trees for operational strategies.
4. Integrate with business systems
Implement predictive analytics within existing business platforms, such as ERP systems, to enable seamless data flow and real-time decision-making.
5. Leverage AI and machine learning
Utilize advanced algorithms to improve prediction accuracy and automate data processing, enabling businesses to make proactive, data-driven decisions.
6. Monitor and refine models
Continuously evaluate the performance of predictive models, update them with new data, and refine algorithms to improve accuracy and relevance over time.
7. Train teams and foster data-driven culture
Equip employees with the knowledge and tools to interpret predictive insights, ensuring that analytics-driven decision-making becomes a core part of business operations.
Enhancing Business Efficiency through Predictive Analytics in HashMicro ERP System
One of the advanced ERP software that provides predictive analytics is HashMicro. With its comprehensive system, HashMicro ERP System helps businesses analyze real-time data, forecast trends, and make proactive decisions to optimize operations.
With over 2,000 clients served, HashMicro provides various advantages to optimize business operations. Plus, you can try it for free with our demo. Below are the key benefits of HashMicro ERP Software.
- Built in business intelligence (BI)
- Whatsapp integration
- Sheet management
- AI integration
- Weather forecast integration
- Available on mobile apps
- Flexible hosting (on-premise/cloud)
- Multi-language (English, Tagalog, Mandarin, Indonesian)
Managing various business processes can be complex without the right tools. HashMicro ERP System offers a comprehensive solution to streamline operations and improve efficiency. Below are the prominent features of HashMicro ERP System:
- Manage thousands of SKUs: Improve precision in handling thousands of SKUs to prevent input mistakes, miscalculations, and inventory loss.
- Smart transaction bookkeeping: Automatically log every expense, cost, bank reconciliation, and sales transaction.
- Sales performance enhancement: Simplify prospect tracking, streamline follow-ups, and accurately forecast sales. Enhance sales team effectiveness.
- Planned stock purchases: Activate automatic buying as stock nears its minimum, promoting seamless transactions.
- Real-time stock opname: Instantly assess stock levels and track real-time product movement.
- Production cost control: Manage production expenses from procurement and output to handling defective components.
Conclusion
Predictive analytics employs statistical methods, machine learning, and historical data to predict future trends and results. This approach enables businesses to foresee potential challenges, enhance decision-making, and boost operational efficiency.
Integrating predictive analytics with an ERP system is beneficial for obtaining real-time data and enhancing decision-making. HashMicro ERP System provides comprehensive solutions leveraging predictive models to analyze trends, optimize operations, and improve business performance.
Book our free demo now to discover how HashMicro can minimize risks, boost efficiency, and drive long-term growth with predictive analysis. Samahan kami sa pagpapalawak ng iyong negosyo!
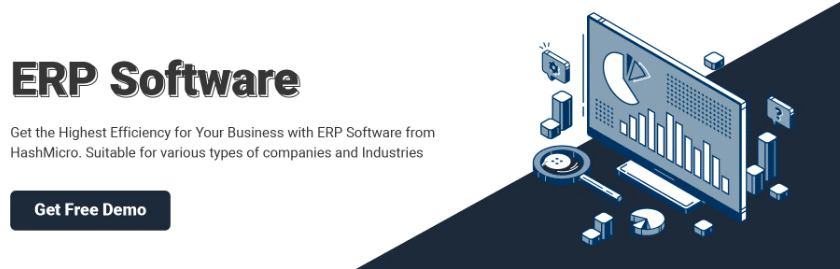
Frequently Asked Questions About Predictive Analytics
-
Is predictive analytics a part of AI?
Machine learning and predictive analytics fall under the broader umbrella of AI, employing its principles in their functions.
-
What is the best way to describe predictive analytics?
Predictive analytics leverages statistical and modeling methods to predict future outcomes. It involves examining and plotting current and historical data patterns to assess the likelihood of their repetition.
-
Is predictive analytics classified as a tool?
Predictive analytics represents one facet of analytics tools. In practical applications, users may not mention the term when engaging with predictive analytics cases.